The goal: Nithio’s goal is to scale capital flows to clean energy solutions. To do so, Nithio has developed its Risk Analytics Engine to standardize credit risk assessments to inform sustainable investment.
The challenge: Credit bureaus are limited and therefore credit risk assessments are largely inaccessible across Africa. This makes it difficult to determine ability and willingness to pay for specific products, including solar products. Moreover, each company collects and reports various metrics with very little standardization. The lack of standardization has in turn stalled capital flows from scaling at the rate needed to reach universal energy access by 2030. With more than 600 million still without reliable energy access, there is a clear need to understand risk within the clean energy sector in order to match the right capital to the right end user to ensure no one is left behind in achieving universal energy access.
So how does Nithio tackle this problem?
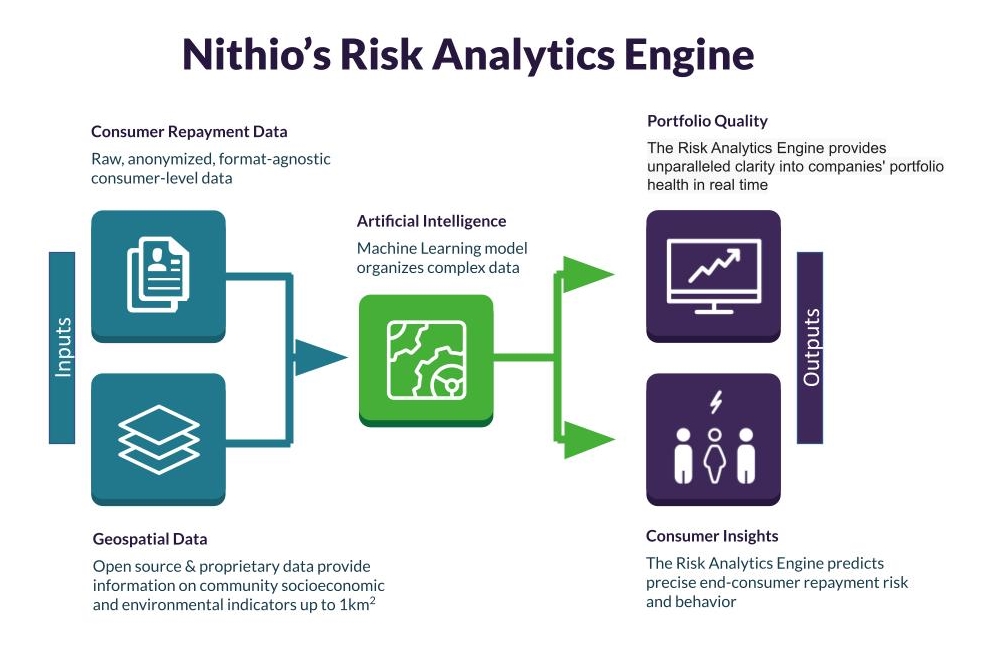
First: Incorporate Geospatial Population Data
At a specific location in the world, geospatial data details various data points, including characteristics of people (demographics), climate (precipitation or rainfall), events (vegetation loss, disease spread) or objects (gas stations, houses). It is a powerful tool for providing insight on population characteristics, dynamics, and behaviors. Researchers at the World Bank, for example, have found that satellite imagery can provide insight on socioeconomic characteristics and poverty levels in the paper “Poverty from Space: Using High-Resolution Satellite Imagery for Estimating Economic Well-Being.”
Nithio incorporates geospatial data from open data sources as well as proprietary data providers, such as Fraym. This data provides hundreds of indicators, including energy access, health and sanitation access, housing quality, consumer power, education levels, employment, across countries or regions down to the 1 square kilometer level. The data is an integral component to understanding consumer characteristics.
Next: Match and Integrate Customer Repayment Data
To assess the credit quality of a company’s customer base, we then look at how their customers have behaved historically. Nithio incorporates raw, anonymized customer-level data from a company to build a standardized credit risk assessment. Nithio’s approach is technology agnostic and can incorporate data from various data management tools, including excel spreadsheets, formalized CRM systems (such as Angaza, PaygOps, and Upya), and internal systems. Once set up, Nithio can get real-time updates about customers’ characteristics and payments.
Historical data can be used in many ways to predict future outcomes. For example, as income relates to consumer ability to repay, we might expect higher income to mean the consumer will likely repay on time. The consumer repayment profile becomes more illuminated when more predictors are added—such as asset ownership, alongside education, family size, consumer prices, oil or gas prices, electricity availability and much more. Nithio leverages Artificial Intelligence to be able to process millions of data points.
Leverage AI to Bring Standardization + Specificity
As both the geospatial data and the customer repayment data are geotagged, Nithio uses both as inputs into its AI models. Nithio’s model is a subset of AI— a Machine Learning (ML) model. The program builds a model based on a sample of historical data (or “training data”), looking for patterns between the inputs and an outcome (such as repayment patterns) that we wish to predict. ML models can capture and standardize across the complexity of massive numbers of data points with highly varied circumstances–and the richer the training set, the better the model. Nithio has built an expansive training data set with millions of localized data points. Our data foundation and uniquely adapted ML methods allow us to iteratively test, validate, and improve our models so we can accurately predict future outcomes for new portfolios of customers.
The Output
Nithio’s innovative approach looks forward, whereas previously the industry was relying only on historical data analysis. Our Risk Analytics Engine predicts customer characteristics from the point of origination, and can accurately forecast repayment rates and future cash flows of companies with an accuracy that far outstrips estimations drawn from historical time series data. The output is a tailored assessment of customers’ credit risk within a specific company, providing integral insight for due diligence, improving companies’ bankability, monitoring portfolio health, and tracking investment impact. This new, innovative credit risk assessment enables investors to sustainably scale their clean energy financing in a standardized approach that hasn’t been possible before.
Recent Comments